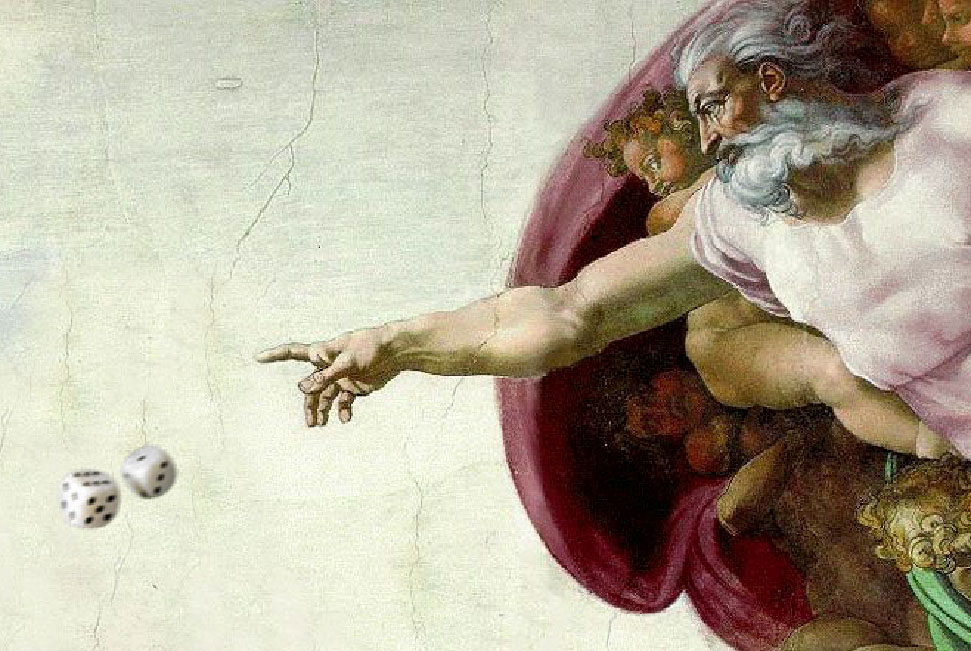
I'm by no means an expert in this field, so my take is going to be less than professional. But my impression is that although the Bayesian/Frequentist debate is interesting and intellectually fun, there's really not much "there" there...a sea change in statistical methods is not going to produce big leaps in the performance of statistical models or the reliability of statisticians' conclusions about the world.
Why do I think this? Basically, because Bayesian inference has been around for a while - several decades, in fact - and people still do Frequentist inference. If Bayesian inference was clearly and obviously better, Frequentist inference would be a thing of the past. The fact that both still coexist strongly hints that either the difference is a matter of taste, or else the two methods are of different utility in different situations.
So, my prior is that despite being so-hip-right-now, Bayesian is not the Statistical Jesus.
I actually have some other reasons for thinking this. It seems to me that the big difference between Bayesian and Frequentist generally comes when the data is kind of crappy. When you have tons and tons of (very informative) data, your Bayesian priors are going to get swamped by the evidence, and your Frequentist hypothesis tests are going to find everything worth finding (Note: this is actually not always true; see Cosma Shalizi for an extreme example where Bayesian methods fail to draw a simple conclusion from infinite data). The big difference, it seems to me, comes in when you have a bit of data, but not much.
When you have a bit of data, but not much, Frequentist - at least, the classical type of hypothesis testing - basically just throws up its hands and says "We don't know." It provides no guidance one way or another as to how to proceed. Bayesian, on the other hand, says "Go with your priors." That gives Bayesian an opportunity to be better than Frequentist - it's often better to temper your judgment with a little bit of data than to throw away the little bit of data. Advantage: Bayesian.
BUT, this is dangerous. Sometimes your priors are totally nuts (again, see Shalizi's example for an extreme case of this). In this case, you're in trouble. And here's where I feel like Frequentist might sometimes have an advantage. In Bayesian, you (formally) condition your priors only on the data. In Frequentist, in practice, it seems to me that when the data is not very informative, people also condition their priors on the fact that the data isn't very informative. In other words, if I have a strong prior, and crappy data, in Bayesian I know exactly what to do; I stick with my priors. In Frequentist, nobody tells me what to do, but what I'll probably do is weaken my prior based on the fact that I couldn't find strong support for it. In other words, Bayesians seem in danger of choosing too narrow a definition of what constitutes "data".
(I'm sure I've said this clumsily, and a statistician listening to me say this in person would probably smack me in the head. Sorry.)
But anyway, it seems to me that the interesting differences between Bayesian and Frequentist depend mainly on the behavior of the scientist in situations where the data is not so awesome. For Bayesian, it's all about what priors you choose. Choose bad priors, and you get bad results...GIGO, basically. For Frequentist, it's about what hypotheses you choose to test, how heavily you penalize Type 1 errors relative to Type 2 errors, and, most crucially, what you do when you don't get clear results. There can be "good Bayesians" and "bad Bayesians", "good Frequentists" and "bad Frequentists". And what's good and bad for each technique can be highly situational.
So I'm guessing that the Bayesian/Frequentist thing is mainly a philosophy-of-science question instead of a practical question with a clear answer.
But again, I'm not a statistician, and this is just a guess. I'll try to get a real statistician to write a guest post that explores these issues in a more rigorous, well-informed way.
Update: Every actual statistician or econometrician I've talked to about this has said essentially "This debate is old and boring, both approaches have their uses, we've moved on." So this kind of reinforces my prior that there's no "there" there...
Update 2: Andrew Gelman comments. This part especially caught my eye:
Update 3: Interestingly, an anonymous commenter writes:
Update 4: A commenter points me to this interesting paper by Robert Kass. Abstract:
Update: Every actual statistician or econometrician I've talked to about this has said essentially "This debate is old and boring, both approaches have their uses, we've moved on." So this kind of reinforces my prior that there's no "there" there...
Update 2: Andrew Gelman comments. This part especially caught my eye:
One thing I’d like economists to get out of this discussion is: statistical ideas matter. To use Smith’s terminology, there is a there there. P-values are not the foundation of all statistics (indeed analysis of p-values can lead people seriously astray). A statistically significant pattern doesn’t always map to the real world in the way that people claim.
Indeed, I’m down on the model of social science in which you try to “prove something” via statistical significance. I prefer the paradigm of exploration and understanding. (See here for an elaboration of this point in the context of a recent controversial example published in an econ journal.)
Update 3: Interestingly, an anonymous commenter writes:
Whenever I've done Bayesian estimation of macro models (using Dynare/IRIS or whatever), the estimates hug the priors pretty tight and so it's really not that different from calibration.
Update 4: A commenter points me to this interesting paper by Robert Kass. Abstract:
Statistics has moved beyond the frequentist-Bayesian controversies of the past. Where does this leave our ability to interpret results? I suggest that a philosophy compatible with statistical practice, labeled here statistical pragmatism, serves as a foundation for inference. Statistical pragmatism is inclusive and emphasizes the assumptions that connect statistical models with observed data. I argue that introductory courses often mischaracterize the process of statistical inference and I propose an alternative "big picture" depiction.
I'm not a statistician either, but as I under it likelihood models are Bayesian models with (invariantly) flat priors, mathematically. Frequentist models are special cases of generalized linear likelihood models. So frequentists are likelihoodists who are Bayesians who a) don't know it; b) implicitly assume that they know nothing about the world when they specify their models (which is self-contradictory).
ReplyDeleteBayesians frequently test their models to see how sensitive they are to the prior, so your concern above is usually not a problem in practice. Bayesians frequently use uninformative priors to "let the data speak", at least as a baseline. The point is that Bayesian models are more flexible, involve more realistic assumptions about the data generating process, yield much more intuitive statistical results (i.e. actual probabilities), and provide much more information about the relationship between variables (i.e. full probability distributions rather than point estimates).
That said, the differences between the two from the perspective of inference are usually minor, as you note.
Hmm, I'm not sure there can exist such a thing as an "uninformative prior"...and sensitivity tests almost certainly only test a limited set of priors, which can lead to obvious problems...
DeleteAs for providing "much more information" with the same amount of data...most of that "information" is actually just going to be the prior.
"Uninformative" in the sense that when a prior is specified in a Bayesian model it is also given a level of confidence. If one wants weak priors -- so weak as to be trivial, even, except perhaps in extreme cases -- one can specify the model that way and still benefit from the other advantages of Bayes.
Deletehttp://www.stat.columbia.edu/~gelman/presentations/weakpriorstalk.pdf
Yes, sensitivity analyses only include a limited set of priors, but frequentist/likelihoodist analysis only has ONE set of priors, which are implicit and atheoretical. Mathematically that's what's happening even if frequentists don't realize it (which they generally don't). Tell me which is more likely to be problematic.
I am a utilitarian about this stuff... I see no reason not to specify the model a variety of ways. If the results diverge sharply then you need to figure out why. If they don't then you can take advantage of the flexibility of Bayes to interpret probabilities directly.
The part about weak priors wasn't phrased as precisely as it could have been, but hopefully you get the idea.
DeleteI think some people support a way of defining 'uninformative prior' in an (arguably) more rigorous way by means of using Lagrange multipliers and maximum information entropy. In brief you seek the distribution with the maximum entropy given constraints such as (at least) the need for the distribution to sum to 1.
DeleteGenerally this procedure gives results that are more or less what you would expect - for example if p is the probability of a coin producing a 'heads' when flipped, the maximum entropy prior is to assume a constant probability density for p between 0 and 1. It turns out that the maximum entropy probability density distribution for the common case where you want at least defined and integrable first and second moments (but not necessarily any more) is Gaussian, which provides a link to frequentist methods.
I think that one reason that Bayesian methods have not become more popular is the computation difficulty of the integrals involved when you get away from toy problems. They can get quite high-dimensional and multi-modal etc. If you use aggressive approximation techniques to attack these integrals you can end up with results that are hard to independently re-create; which may make it hard to analyse errors; which may be unstable to changes in techniques, coding or parameters; or which may have made subtle assumptions (such as fitting Jacobians to modes) that could make the process dubious.
Noah, please google 'uninformative prior'.
Delete"
(note - copy and paste with an iPad doesn't work)
DeleteNoah, the example Cosma gives isn't a failing of bayesian technigues, but rather the fact that a bad model gives bad results, no matter how much data one has.
The statement that frequentist statistics (maximum likelihood) is the same as Bayesian with a flat prior, that's true only is specific textbook examples, and only if the supposed Bayesian reports only the posterior mean.
DeleteA significant difference between Bayesian and frequentist statistics is their conception of the state knowledge once the data are in. The Bayesian has a whole posterior distribution. This describes uncertainies as well as means.
I think it is pretty indisputable that the Bayesian interpretation of probability is the correct one. Probability measures a degree of belief, not a proportion of outcomes.
ReplyDeleteP*≠P
The observed probability distribution (P) does not equal the real probability distribution (P*). In the nonlinear and wild world we live in, only continued measurement into the future can give us P* for any future period (generally, we underestimate the tails). In an ultra-controlled non-fat-tailed environment P can look a lot like P* (making the frequentist approach look correct) but even then P* may diverge from P given a large enough data set (one massive rare event can significantly shift tails).
Why does the frequentist approach survive? Because it is useful in controlled environments where black swans are negligibly improbable. But it should come, I think, with the above caveat.
No, the Bayesian interpretation of probability is obviously ridiculous. "Degree of belief" is just some mumbo-jumbo that Bayesians like to say like it means anything. When I say something has 50% probability, then I definitely think that if you do it over and over again, it will happen 50% of the time. It's a statement about long-run averages that it meant to be objective.
DeleteNothing you said refuted anything I said. Frequentism pretends to be objective, and it really isn't because humans are subjective creatures with priors. Bayesianism is at least honest about its priors.
DeleteI agree with walt. When a Bayesian talks about "real probability distribution", and "continued measurement", he/she IS a frequentist, at least a frequentist in my understanding.
DeleteOne impression of mine is that the Bayesians tend to be more aggressive than the frequentists, and frequentists tend to talk in a humble way.
This is understandable, since, as the author of this blog (Smith) said in the post, the Bayesian is an approach which is likely to be used when the data is not great. It is no surprise that a person who is trying to "get something from nothing" tends to be ambitious, and, aggressive.
But ultimately I guess there might be some differences in the brain structure between the frequentists and Bayesians. Anyone interested in testing this, using MRI for example?
The basic philosophy of Bayesianism seems to appeal to me more, just because you have to put your prior assumptions out there. Most of the critique that I've seen comes from having intentionally stupid priors, but questioning assumptions should be a big part of modeling.
ReplyDeleteI dont know how noah smith can think he is an economist... its so embarrasing, please bury yourself or put glue in your mouth
ReplyDeleteOK, will do...
DeleteNo, dude, don't do that! That might kill you!
DeleteI think you've missed an important point about Bayesian statistics--essentially, choosing a prior lets the statistician to formally incorporate information we already know into the analysis. This prior knowledge could come from other research papers on the topic, prior stages in the same experiment (very useful in clinical trials) or maybe just intuitive logic. Frequentists do exactly the same thing, but the difference is that they aren't supposed to--it technically invalidates their results. Consider for example a placebo-controlled clinical trial. The treatment and placebo groups are never truly random--ethics dictate that we balance the two groups to look as similar as possible, because this will increase the statistical power and help us identify potential risks of treatment much sooner, potentially saving lives. At the end of the trial we get a frequentist p-value of, say, 0.05, but in reality this is wrong--we are pretty sure that because of balancing the two groups the real p-value is less than 0.05, but a frequentist has no way of knowing what it actually is. My understanding is that in Bayesian statistics this is no longer a concern--we know that our results are accurate given all the available information, including both the prior and the data.
ReplyDeleteAlso, I mentioned the need for a clinical trial to do ongoing statistics to identify risks to the treatment group as soon as possible. Strictly speaking, this ongoing analysis also invalidates the frequentist results--continuation of the trial should be completely independent of the results of the trial, and not shut down when we have evidence of harms to the patients. Bayesian statistics, by contrast, allows us to do ongoing analysis without in anyway invalidating the results. And since Bayesian inferences incorporate both the prior information and the data, it can statistically identify risks to patients in the trial much sooner than can frequentist methods. In that respect, it could actually be considered unethical to rely on frequentist methods for human-subjects research that involves more than minimal risk.
More generally, I think the point needs to be made that frequentist probability theory is really just a subset of Bayesian theory but with lots of implicit assumptions about the prior that aren't necessarily justifiable.
That said, you are right--Bayesian statistics won't be able to tell us a whole lot that we didn't already know. For the most part it tells us the same things but with a purer internal logic.
"formally incorporate information we already know"
DeleteThat's nice and everything, but what's unclear to me is how our prior knowledge, usually vague and diffuse (otherwise there would be no point in further analysis), is supposed to translate into precise distributions with precise parameters. Is it just a coincidence that Bayesians always use same few well-known textbook distributions (gaussian, beta, gamma...) for their priors?
Of course, those particular priors are approximations used for analytic convenience, and that's fine, but it still kind of subtracts from advantages of "purer internal logic". Bayesian paradigm is a nice model of how people update beliefs, in the same sense as utility maximization is a nice model of how people decide what to buy - but it's not obvious to me whether we really should take it literally (just as we don't literally solve constrained optimization problems while shopping).
As for balancing control and treatment groups, it's not like people are unaware of it. Searching for "stratified randomization" yields quite a few results.
If you weaken your priors due to lack of evidence, I posit you are a Bayesian. The Frequentist takes his hypothesis and data as fixed. If he chooses to alter them, he is doing another experiment. In this, a Bayesian is just a Frequentist doing multiple experiments in succession, often on the same data, whereas the Frequentist would be concerned the change in hypothesis might invalidate the data collection.
ReplyDeleteMinor point: I've heard that one reason Bayesian statistics hasn't been used a lot more in economics is simply because, until the last 20(?) years or so, it was very hard to implement computationally. The falling cost of computing power has really opened things up now, because (I think) you can implement a lot of analytically intractable stuff numerically or by simulation. Now there's a bit of slow adjustment going on, as people trained in frequentist methodology update their skill sets. Anyway, that's what they're telling me in some of my econometrics classes. So, maybe Bayesian statistics hasn't had really had several decades to try and prove its superiority.
ReplyDeleteThat said, it has seen a lot of use in the past decade, and I agree with you that it doesn't seem to be a game-changer.
I'm sure that's true for sophisticated statistical models but not all Bayesian statistics require difficult computation. My undergrad probability theory professor forced us to crank out some basic Bayesian statistics by hand on exams.
DeleteI'd say one reason Bayesian statistics isn't popular in economics could also be that it is already a profession plagued by too many subjective biases. Since the field has political ramifications, economists want to shield their results from charges that they chose politically motivated priors. And invariably there will be some econ papers that actually do choose politically motivated priors...
I'd say one reason Bayesian statistics isn't popular in economics could also be that it is already a profession plagued by too many subjective biases. Since the field has political ramifications, economists want to shield their results from charges that they chose politically motivated priors. And invariably there will be some econ papers that actually do choose politically motivated priors...
DeleteThis rings true to me...very perceptive...
Noah,
ReplyDeleteThere are at least two kinds of debate that look the same but are not. The philosophical Bayesian x Frequentist and the practical silly "Null Hypothesis Significance Testing (NHST)" x "Please, think about what the hell you're doing".
Nate Silver points most to the second debate. When he says frequentism, he is really saying silly NHST. Of course, some people get mad with that, because they claim the name "frequentist" to themselves and do not like when bad practice is associated with that name.
Now, why it is important to state the difference between this two debates?
Take your statement for example: "When you have a bit of data, but not much, Frequentist - at least, the classical type of hypothesis testing - basically just throws up its hands and says "We don't know.""
That is not true in practice.
When you have a bit of data, you usually do not reject the Null Hypothesis. And what do people do? They don't say "we don't know", they say that there is evidence in favor of the null, whithout ever checking the sensitivity (power or severity) of the test (needed in a coherent frequentist approach), nor, in Bayesian terms, the prior probability of the hypothesis...
So both would make an statement about reality with crappy data.
When you have a bit of data, you usually do not reject the Null Hypothesis. And what do people do? They don't say "we don't know", they say that there is evidence in favor of the null, whithout ever checking the sensitivity (power or severity) of the test (needed in a coherent frequentist approach)
DeleteYes. This is a very bad way to do things, just as it is bad in Bayesian to start with an extremely strong prior and conclude in favor of that prior. In fact it's a very similar mistake.
Posting the comment that I have posted on Brad DeLong's:
ReplyDeleteWhen you see people doing significance testing in applied work, how often do you see they stating the sensitivity (power or severity) of the test against meaninful alternatives? (I'll answer that... from 80 to 90% do not even mention it, see: http://repositorio.bce.unb.br/handle/10482/11230 (portuguese) or McCloskey "The Standard Error of Regressions")
This is not because there are not papers teaching how to do it (at least approximately): e.g. see Andrews (http://ideas.repec.org/a/ecm/emetrp/v57y1989i5p1059-90.html)
And there are plenty of papers with meaningless debates going on with underpowered test, for example, the growth debate arround institutions x geography x culture...
Noah,
ReplyDeleteI would appreciate your moving from the abstract to the specific.
Slackwire has up a very interesting graph, on the decline in bank lending for tangible capital/investment?
http://slackwire.blogspot.com/2013/01/what-is-business-borrowing-for.html
You have written about the economy breaking in the early 1970s, which the graph confirms to my eye.
Is this graph Bayesian/Frequentist or Delong's third way or something else entirely
If the probability of a hypothesis being true given the data is what you want to know, then presenting anything else (e.g. the probability that your data would be different if an alternative hypothesis were true) isn't being frequentist so much as it is being a bad statistician. However in a lot of cases neither of these questions are precisely what matters and you are really using statistics to get at something a little fuzzier -- have I collected enough data, am I taking reasonable steps to prevent myself from seeing patterns in noise, etc. Often when testing scientific hypotheses the precise probability is uninteresting but a significance test is important, and here either approach can help. In fact you would not want to publish a result that passed a bayesian test but failed a frequentist test, not because the conclusion is particularly likely to be wrong but saying "my experiment doesn't add much certainty but given what we already know the conclusion is quite certain" is not an interesting result.
ReplyDeleteI don't agree with this, though:
It seems to me that the big difference between Bayesian and Frequentist generally comes when the data is kind of crappy.
The difference also comes when the data on priors is good, and especially when the prior is lopsided. The latter may sound like a corner case, but it is the normal case in medicine and there are plenty of other cases in the real world. I (well, not me exactly) had a health scare recently, and it would have seemed much worse had we been presented with inappropriate frequentist statistics (the false positive rate of this test is low, so null hypothesis rejected with high confidence!) rather than the prior and posterior probabilities we were presented with (In the absence of this test you would be very unlikely to have this; given the result there is a 1 in 30 chance you have this.) Of course, DeLong's "value of being right" considerations applied here and we opted to do further testing to make sure we were fine, but it goes to show the difference can be big even with good data.
That seems right to me. Although I'm not doing those sorts of analysis much anymore so I'm not sure my view's worth much. Seems to me in practice most scientists practice a staggering array of inconsistency when it comes to either epistemology or metaphysics. (And of course one needn't engage in the philosophical debate here) So just as say a typical physicist engages in an incoherent mix of instrumentalist, empirical and realist approaches to physics I suspect the average science (I'll even throw economists in there) engages in a mix of Bayesian and Frequentist approaches. As you say, often those more sympathetic to Frequentist approaches simply weaken their priors. At least that's what I see in practice although not always what is argued for.
ReplyDeleteMy old adviser, Chris Lamereoux, was a big Bayesian, with some well known Bayesian papers. I talked with him about this years ago, about the obviousness of including important prior information, and he said the smart sensible thing; good frequentest statisticians and econometricians of course consider apriori information, but do so in an informal and less rigid way.
ReplyDeleteLet Pr(H) be our degree of belief in hypothesis H; Pr(E) our degree of belief in evidence E. Suppose we perform experiments that repeatedly demonstrate E. We may model this as Pr(E) -> 1.
ReplyDeleteRecall that Pr(H) = Pr(H|E)*Pr(E) + Pr(H|~E)*Pr(~E) [the law of total probability, restated in terms of conditional probabilities]. As Pr(E) -> 1, Pr(~E) -> 0, and Pr(H|~E) -> 0. Thus, Pr(H) -> Pr(H|E). In short, as we become very nearly certain of E, our degree of belief in H ought to condition on E.
Frequentists don't really have grounds for disagreeing with the above. Most frequentist procedures can be defended on Bayesian grounds, provided the appropriate loss function and prior, so you're correct that this is not a major practical issue for statisticians. The problem occurs when you're trying to teach a computer to learn from its observations. The only way to do frequentist inference sensibly is to implicitly be a reasonable Bayesian. Without making this explicit, though, a computer is not going to do frequentist inferences sensibly without a human going through its SAS output or the like.
That's nice and everything, but what's unclear to me is how our prior knowledge, usually vague and diffuse (otherwise there would be no point in further analysis), is supposed to translate into precise distributions with precise parameters.
ReplyDeleteBINGO. The practical differences are more political than anything else, possibly because Bayesians suffer from what I'll call 'Frequentist envy'. We've all seen this one a million times: some study that claims statistical significance with p set at - surprise! - 0.05. Queue much gnashing of teeth from the stat folks about how this runs against the grain of good statistical practices. Then the Bayesians jump in and start sneering about this thing called 'priors'. 'Priors'? Do you mean the application of domain-specific knowledge that could just as easily been done with the old way, and should have been? That's some mighty thin gruel there, yet that's what the debate really seems to come down to so often in practice.
Frequentists are well aware of Bayes theorem, btw, and use it quite routinely. Something that Bayesians like to pretend doesn't happen all that often. And most people I know use Bayesian methods when the situation calls for it and Frequentist methods likewise. They're just tools in the toolbox after all.
Ack, I tire of this old debate too. And I agree that there is far more heat than light to be found in it.
ReplyDeleteBut you do kind of walk all over the underlying philosophies, as well as some practical issues.
A few points:
1.) Frequentists and Bayesians may have nearly identical uncertainty measures in most cases but they interpret them differently. This would see to have little practical difference in how the measures are applied.
2.) philosophical frequentists hate it when anyone uses their uncertainty measures as if they are Bayesian measures (which is typical); the converse is not true.
3.) Bayesian tools, like Makov Chain Monte Carlo, can be useful even if you're a frequentist at heart.
When I don't know my audience I go for the low-brow approach (not wrong, not confusing, and hopefully, not insulting), which is the case here.
ReplyDeleteSo looking back over the comments, I think Carlos nails it at comments 8:18 and 8:45.
Yes, there are significant differences between the two approaches, and yes, there are times when one would clearly prefer one over the other. But 95% of the time what you see is meaningless bickering ;-) The Nate Silver thing falls into that 95%. Imho.
For me the reason for the Bayesian surge has been computers. In my job as a Bioinformatician, I built a Bayesian generative model, the results of which are sold for 70 dollars a pop wholesale. In 2007 when I finished the work it took 15 minutes to run. This wouldn't have been possible with Bayes's own calculating methods (paper and pen).
ReplyDeleteThe main reason for the extensive computer involvement is the calculation of likelihoods based upon multiple non-independent data sources that can't be dealt with linearly. So hidden-markov-models mixed with generative elements produce a far superior result.
None of this would be possible with just frequentist stats.
So I say that the reason for the rise of the Bayesians isn't some shift in opinion about how science or statistics should be done but that it's the only proper way to use the incidental data we have and it's only recently that we can actually succeed.
I think a key missing element was discovered by Mandelbrot with his research on fractals, which I will get to. But first what if the events cannot be precisely measured? In this case a frequentist interpretation of “proof” is in principle impossible, and we then become Bayesian using subjective data and whatever additional data which we 'deem' relevant to elements of the analysis to form a “prior.” In a review of Mandelbrot’s The Misbehavior of Markets, Taleb offers an interesting formula that he says: “seems to have fooled behavioral finance researchers.” He writes: “A simple implication of the confusion about risk measurement applies to the research-papers-and-tenure-generating equity premium puzzle. It seems to have fooled economists on both sides of the fence (both neoclassical and behavioral finance researchers). They wonder why stocks, adjusted for risks, yield so much more than bonds and come up with theories to “explain” such anomalies. Yet the risk-adjustment can be faulty: take away the Gaussian assumption and the puzzle disappears. Ironically, this simple idea makes a greater contribution to your financial welfare than volumes of self-canceling trading advice.” The pdf of the review is here (http://www.fooledbyrandomness.com/mandelbrotandhudson.pdf)
ReplyDeleteSo my question is should we move beyond Bayesian and Frequentist when looking at probabilities and look at fractals otherwise we omit what Mandelbrot called “roughness.” In other words research focuses too much on smoothness, bell curves and the golden mean and if we look at roughness in far more detail will we will be able to provide greater insight to the matter at hand?
I really think people should take a look at this Chris Sims' text, found at http://sims.princeton.edu/yftp/EmetSoc607/AppliedBayes.pdf
ReplyDeleteAlso, the open letter by Kruschke is worth your while:
http://www.indiana.edu/~kruschke/AnOpenLetter.htm
"Basically, because Bayesian inference has been around for a while - several decades, in fact"
ReplyDeleteHow about centuries? ;)
The frequentist view was a reaction against the Bayesian view, which came to be perceived as subjective. What we are seeing now is a Bayesian revival. Since this is an economics blog, let me highly recommend Keynes's book, "A Treatise on Probability". Keynes was not a mainstream Bayesian, but he grappled with the problems of Bayesianism. Because the frequentist view was so dominant for much of the 20th century, there is a disconnect between modern Bayesianism and earlier writers, such as Keynes. From what I have seen in recent discussions, it seems that modern Bayesians have gone back to simple prior distributions, something that both Keynes and I. J. Good rejected, in different ways. Perhaps we will see some Hegelian synthesis. (Moi, I think that we will come to realize that neither Bayesian nor Fisherian statistics can deliver what they promise.)
Min is right. Bayesian probability has been around formally for at least 350 years, and the philosophical idea since before the days of Aristotle.
ReplyDeleteI can tell you from experience that Bayesian probability is way more important in the areas of quality control and environmental impact measurements. Noah touches on the reason. You can't assume your process is unchanging or that new pollutants haven't entered the environment. You have to assume they can and eventually will. Essentially, every sample out of spec has to be treated as evidence of a changed process.
I liked Nate Silver's book. An unexpectedly good read. I received the book as a gift and didn't buy it myself, and I expected it to be a dry read, but it wasn't at all.
ReplyDeleteI think the book has the potential for a broad appeal, and when you consider the fact that books that revolve around topics like statistical analysis and Bayesian infeference are usually pompous, inaccessible, and dull beyond belief, I think Nate should be commended for writing something that makes these concepts accessible to a wide audience.
Noah, statistics is not science, they are lies, the worst kinds of lies (Mark Twain: lies, damn lies, and statistics).
ReplyDeleteSomeone above, praising the Bayesian view, forgot to read the 2007 prospectus, which actually reads:
"2 Recent Successes
Macro policy modeling
• The ECB and the New York Fed have research groups working on models using a Bayesian approach and meant to integrate into the regular policy cycle. Other
central banks and academic researchers are also working on models using these methods
http://sims.princeton.edu/yftp/EmetSoc607/AppliedBayes.pdf, page 6
Thank you very much but that track record says it all about statistics. No thanks.
From the entire Western experience, statistics show only one thing about statistics---that statisticians lie, always selecting what they count, blah, blah, blah and how they analyze such to come up with the conclusion they were determined to reach
Statistics are not science yet scientists use this unscientific technique to test their theories as part of the scientific method. That makes no sense but, hey, if Mark Twain said so, it must be true!
DeleteNoah, probably repeating some other comments, but here goes.
ReplyDeleteFirst, Bayesian methods have been around a long time but only recently have emerged because computing costs have come down. It would be interesting to see whether there are now proportionally more papers using Bayesian methods since computing cost went down and if that trend is increasing or stabilizing.
Second, what Matthew Martin said above. Frequentist effectively do the same thing as Bayesians, but pretend otherwise. they build empirical models and throw variables in and out based on some implicit prior which never get reported in their write ups.
Third, my understanding is that Bayesian models generally provide better forecast than frequentist's models. (Not that either are spectacular).
David, my understanding is that the variables frequentists throw in come, or at least should come, from a consistent theoretical model. Do Bayesians provide a justification for their priors? Also, in your opinion, are the forecasts of the Bayesiand models better enough to justify the computational costs?
Delete"Frequentist effectively do the same thing as Bayesians, but pretend otherwise. they build empirical models and throw variables in and out based on some implicit prior which never get reported in their write ups."
DeleteThis is what I understood to be one of Silver's main points: be up front about your "priors," assumptions and context. Kind of like when bloggers and/or journalists say "full disclosure." The history is that the Frequentist tradtion wanted to be more mathematical and objective, whereas the Bayesians argued that one always has biases and it's better if you are conscious of what they are.
CA, you are correct that they should come from a theory, but in practice--I speak from experience--it is never that clean. Here is another way of seeing this. There are some empirical studies whose results fit a nice story, but whose results upon closer inspection are not robust (e.g. throw in an extra lag or drop one variable and results fundamentally change). How long did these authors work to make their empirical model turn out just right so they could publish a great finding? These authors are effectively just finding through trial and error empirical results to fit their own "priors". At least Bayesians are being upfront about what they are doing. This is what appeals to me about the Bayesian approach.
DeleteI don't know enough to answer your second question, but my sense is that computational costs are now very low for doing Bayesian forecasts. For example, though I generally work with frequentist time series methods I can easily set up a Bayesian vector autoregression using RATS software
"How long did these authors work to make their empirical model turn out just right so they could publish a great finding?"
DeleteDavid, yes, I agree. I too am guilty of trying to find if any specification would work. BUT, when I write the paper I present all reasonable specifications based on theory. And if the results change when, say, I drop a variable, I try to explain why (e.g. that I only have few observations and the variable I dropped is correlated with another). In other words, I present the information and let the reader decide. Yet despite trying to be thorough, I still usually get suggestions from referees asking about this or that. If I add a lag, the referee wants to know why. If I detrend, the referee wants to know why I used this filter and not a different one. It is near impossible to publish in a decent journal if you have only tried a couple of specifications (even though you may present only a couple if your results are robust).
Forgot to mention that, however, referees do "demand" more robustness tests when the results go against their prior (e.g. against what theory predicts). So I am somewhat sympathetic to what you are saying.
DeleteCA,
DeleteWhat you said, about referees challenging your assumptions, asking "what about this?" and "what about that?" rings true to me. You have experienced it as part of what sounds like responsible peer review of scholarly articles. I have experienced exactly what you describe when presenting my statistical analysis and recommendations for business and manufacturing work. If my results are contrary to precedent, I need to be prepared with examples of different specifications that I used, to establish robustness. Or be willing to run this or that to convince others that my findings are valid. Subject matter knowledge helps a lot, to be able to respond effectively. Yes, I realize that what I just said seems to justify suspicion of frequentists, and validate a Bayesian approach! But that will remain a problem, whether Bayesian or frequentist, when applying quantitative analysis to human behavior, especially complex dynamic systems.
There seems to be an underlying mistrust of statistical analysis expressed in some of these comments e.g. the Mark Twain quote, or even the supposedly greater honesty of Bayesians and their priors. Statisticians are not inherently deceitful, nor are we practitioners of pseudo-science. I've used probability models for estimating hazard rates, and time to failure, for mobile phone manufacturing (Weibull distribution, I think). It works. Or rather, it is sufficiently effective to be useful in a very practical way.
Economic models are a different matter than cell phones or disk drive reliability. Exogenous influences are important, and human behavior is difficult to quantify.
Scanned quickly to see if some one commented on your picture at the beginning. Ha Ha, In The Beginning! My reptile brain rang up Einstein immediately and his comment that God does not roll dice.
ReplyDeleteI don't know jack about statistics. Is God a Bayesian or a frequentist?
Whenever I've done Bayesian estimation of macro models (using Dynare/IRIS or whatever), the estimates hug the priors pretty tight and so it's really not that different from calibration.
ReplyDeleteMinor technical point on discussion of Shalizi. Infinity can actually make things worse for Bayesians, particularly infinite dimensional space. So, it is an old and well known result by Diaconiis and Freedman that if the support is discontinuous and one is using an infinite-sided die, one may not get convergence. The true answer may be 0.5, but one might converge on an oscillation between 0.25 and 0.75, for example using Bayesian metnods.
ReplyDeleteHowever, it is true that this depends on the matter of having a prior that it is "too far off." If one starts out within the continuous portion of the support that contains 0.5, one will converge.
To deal with infinities Jaynes recommends starting with finite models and taking them to the limit. There is wisdom there. :)
DeleteIn practice, everyone's a statistical pragmatist nowadays anyway. See this paper by Robert Kass: http://arxiv.org/abs/1106.2895
ReplyDeleteThanks for the post. As a statistician, I think it's nice to see these issues being discussed. However, I think a lot of what has been written both in the post and in the comments is based on a few misconceptions. I think Andrew Gelman's comment did a nice job (as usual) of addressing some of them. To me, his most important point, and the one that I would have raised had he not done so, is this:
ReplyDelete"...non-Bayeisan work in areas such as wavelets, lasso, etc., are full of regularization ideas that are central to Bayes as well. Or, consider work in multiple comparisons, a problem that Bayesians attack using hierarchical models. And non-Bayesians use the false discovery rate, which has many similarities to the Bayesian approach (as has been noted by Efron and others)."
The idea of "shrinkage" or "borrowing strength" is so pervasive in statistics (at least among those who know what they are doing) that it frequently blurs practical distinctions between Bayesian and non-Bayesian analyses. A key compromise is empirical Bayes procedures, which is a favorite strategy of some of our most famous luminaries. Commenter Min mentioned a "Hegelian synthesis." Empirical Bayes is one such synthesis. Reference priors is another.
Which brings me to another important point. In the post and in the comments, it is assumed that priors are necessarily somehow invented by the analyst and implied that rigor in this regard is impossible. This is completely wrong. This is a long literature on "reference" priors, which are meant to be default choices when the analyst is unwilling to use subjective priors. An overlapping idea is "non-informative" priors, which are non-informative in a precise and mathematically well-defined sense (actually several different senses, depending on the details).
Also, I want to note that it can be proven that Bayes procedures are provably superior to standard frequentist procedures, even when evaluated using frequentist criteria. This is related to shrinkage, empirical Bayes, and all the rest. Wikipedia "admissibility" or "James-Stein" to get a sense for why.
Finally, the statement, "If Bayesian inference was clearly and obviously better, Frequentist inference would be a thing of the past," misses a lot of historical context. Nobody knew how to fit non-trivial Bayesian models until 1990 brought is the Gibbs sampler. This is not a matter of computing power, as some have suggested -- the issue was more fundamental.
The great Brad Efron wrote a piece called "Why isn't everyone a Bayesian" back in 1986. Despite not being a Bayesian, he doesn't come up with a particularly compelling answer to his own question (http://www.stat.duke.edu/courses/Spring08/sta122/Handouts/EfronWhyEveryone.pdf). One last bit of recommended reading is a piece by Bayarri and Berger (http://www.isds.duke.edu/~berger/papers/interplay.pdf), who take another stab at this question.
One area where the "crappy data" issue becomes extremely important is in pharmaceutical clinical trials. People tend to think that there are two possible outcomes of trials: a) the medication was shown to work or b) the medication was shown to be ineffective. In fact, there is a third possible outcome: c) The trial neither proves nor disproves the hypothesis that the drug works. In practice, outcome (c) is very common. For some indications, it is the most common outcome.
ReplyDeleteThis leads to charges that pharma companies intentionally hide trials with negative results. They don't publish all their trials! But it turns out to be really hard to get a journal to accept a paper that basically says, "we ran this trial but didn't learn anything."
I forget the exact numbers, but for the trials used to get approval of Prozac, it was something like 2-3 trials with positive outcomes, and 8-10 "failed trials," ie trials which couldn't draw a conclusion one way or the other. This is common in psychiatric medicine. Its hard to consistently identify who has a condition, its hard to determine if the condition improved during the trial, and many patients get better on their own, with no treatment at all (at least temporarily).